Unaddressed Deficits in Current Artificial Intelligence Functions
Artificial Intelligence (AI) is advancing exponentially, pushing the boundaries of what was once thought achievable. The latest innovation in this field is reasoning models based on inference-time compute, offering a new realm of possibilities for AI capabilities.
Sam Altman, OpenAI's CEO, recently wrote, "Systems that point to AI Generalization (AGI) are coming into view... We can now imagine a world where we cure all diseases and can fully realize our creative potential." Anthropic CEO Dario Amodei, on the other hand, stated, "What I've seen inside Anthropic and out over the last few months has led me to believe that we're on track for human-level AI systems that surpass humans in every task within 2-3 years."
However, today's AI lacks a fundamental capability: the ability to continue learning. Despite the current approach being universal and entrenched, this shortcoming is hindering true human-level AI. To put it simply, AI can't learn as it goes and adapt to changing circumstances.
Today, AI systems go through two phases: training and inference. During training, an AI model learns from a dataset, and in inference, it generates outputs and completes tasks based on what it learned during training. Unfortunately, an AI's learning only happens during the training phase. After training is complete, the model's weights become static, making it incapable of incorporating new information on the fly to continuously improve itself.
The concept of eliminating the rigid distinction between training and inference, enabling AI systems to learn continuously like humans, goes by many names, including continual learning, lifelong learning, incremental learning, and online learning. It has long been a goal of AI researchers, but its achievement still seems elusive.
Recently, the term 'test-time training' has emerged, referring to updating the model's weights to learn new things or absorb a substantial amount of new context. Perplexity CEO Aravind Srinivas commented, "Going to be amazing when that happens."
Untangling the fundamental problems of continual learning will result in profound implications for the world of AI. But research is progressing, with startups and research labs making exciting advancements in this field.
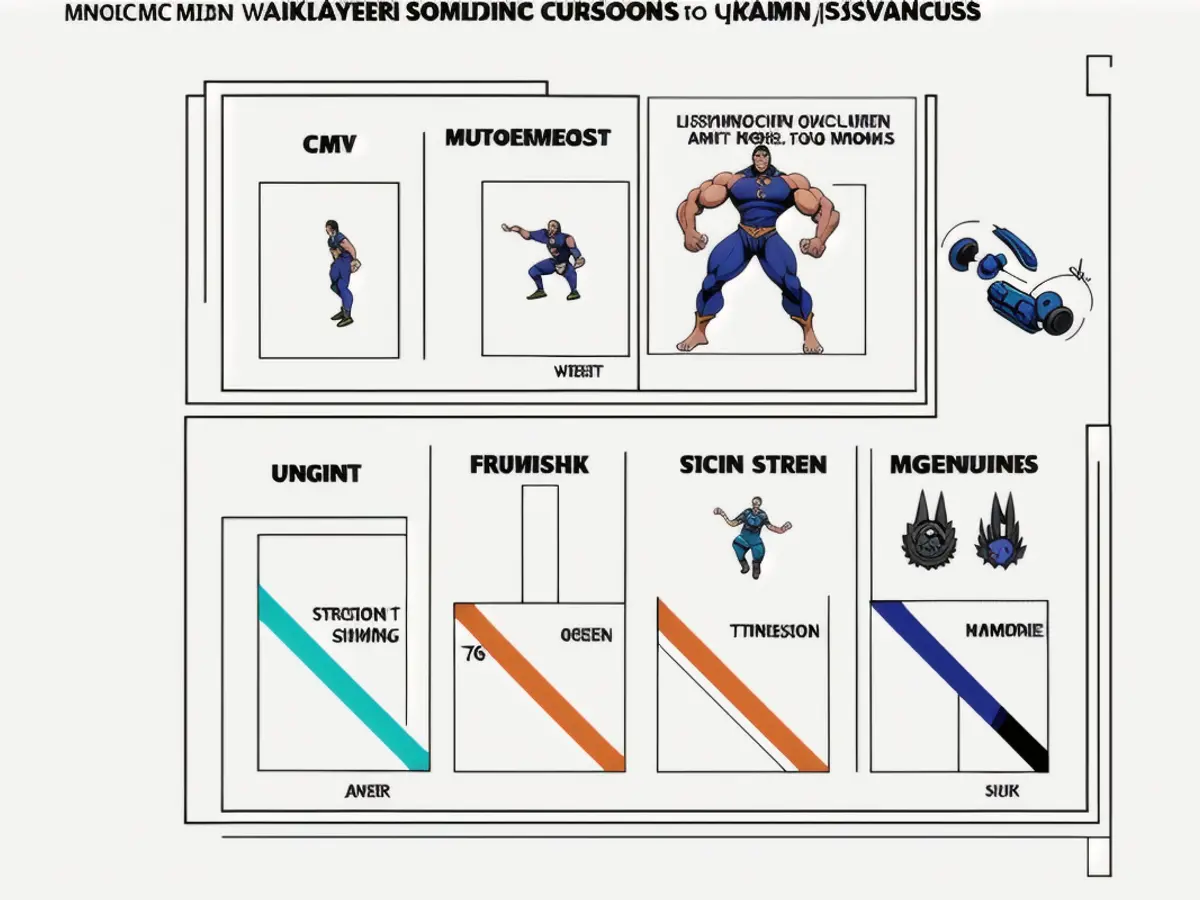
Although workarounds exist, such as model fine-tuning, retrieval augmented generation paired with a dynamically updated vector database, and in-context learning, none fully solves the problem. These methods offer partial solutions but do not unlock true on-the-fly learning.
In the future, continual learning could create durable moats for the next generation of AI applications. As a user interacts more with an AI model, the model becomes more tailored to their context, use cases, preferences, and environment.
Imagine a personal AI agent that adapts to your unique needs and idiosyncrasies in real-time, fostering an enduring relationship with you. This level of personalization will make AI products sticky in ways never seen before, increasing retention and customer loyalty.
There is still much work to be done to make continual learning a reality, but the possibilities are vast and exciting. The application of AI in its continually learning form will revolutionize the consumer and enterprise sectors alike. Stay tuned for this rapidly evolving field and the incredible developments that lie ahead.
Enrichment Data: Continual Learning represents a significant advancement in AI, wherein AI systems can learn and adapt without requiring significant retraining. This flexibility will enable AI systems to better address catastrophic forgetting, a prominent challenge in AI development. However, challenges such as data quality, scalability, talent gaps, and infrastructure constraints need to be addressed for continual learning to reach its full potential.
- Prudential concerns about the integration of test-time training into AI systems are justified, as it could potentially jeopardize the progress of startups working on continual learning research.
- Highlighting the importance of continual learning, Sam Altman's vision for AI suggests that its implementation could lead to the cure of all diseases and the realization of our creative potential.
- The advancement of continual learning in AI, through efforts from startups and research labs, could ultimately highlight the transformative impact on industries, creating lasting bonds between users and AI agents, akin to personal AI assistants that learn and adapt to an individual's unique needs in real-time.